Article
Mastering rail data complexity
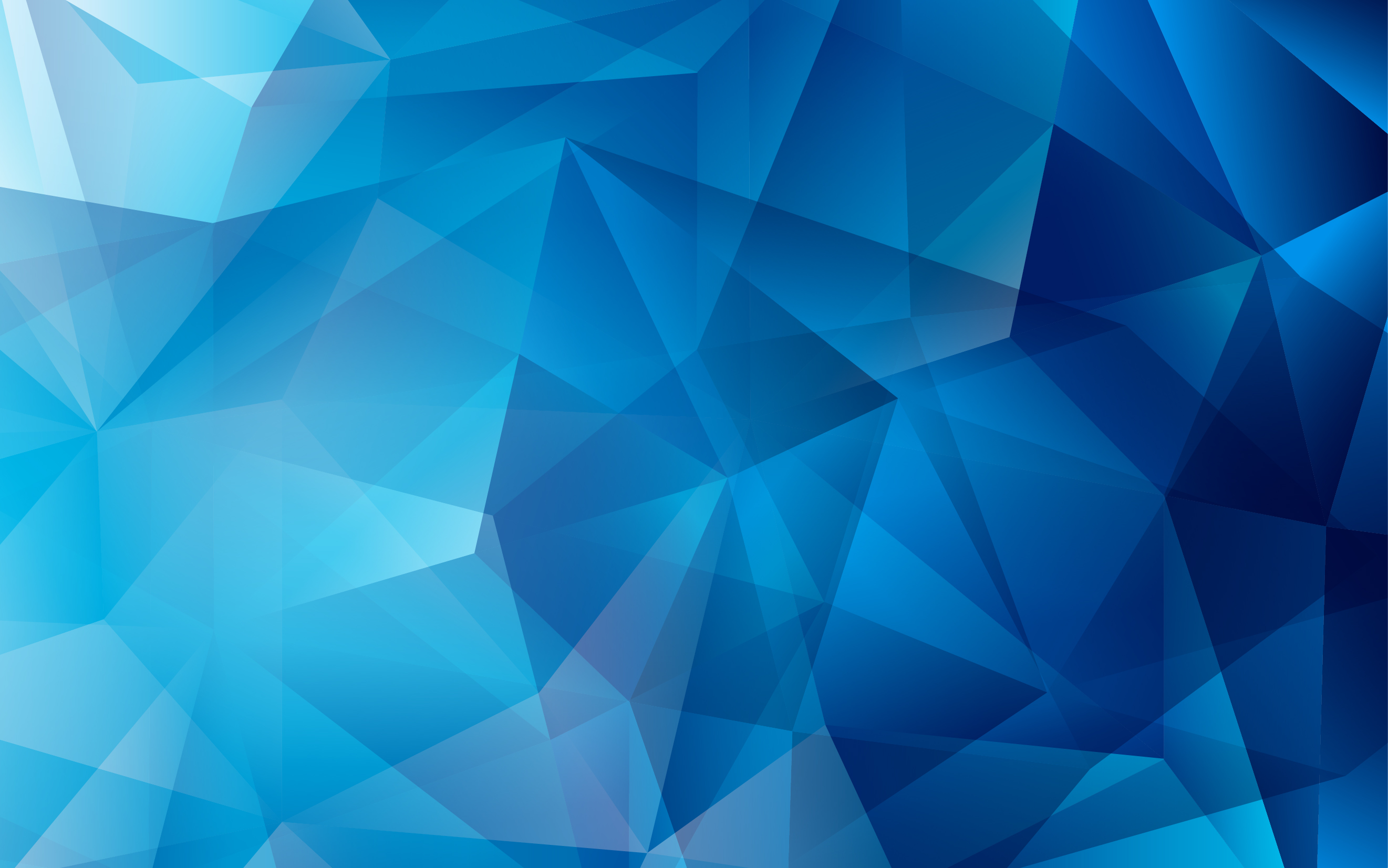
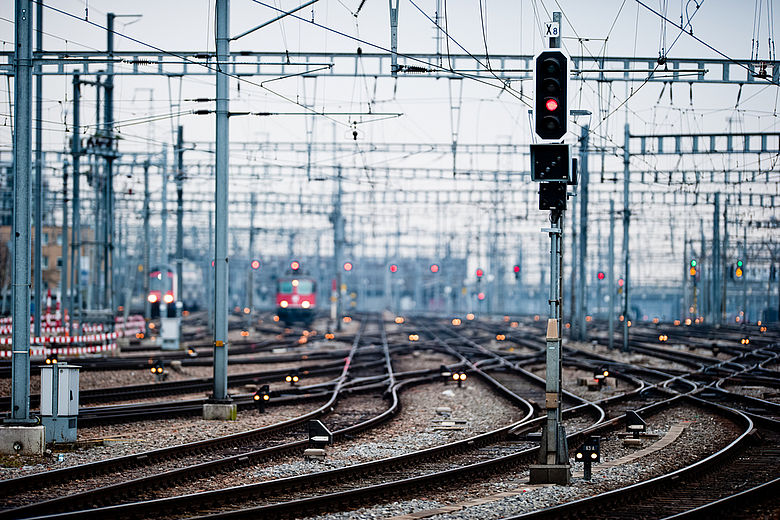
The rail operations ecosystem is a complex puzzle. Historically grown over decades, the system and its assets today are highly heterogeneous. The efficiency of every asset within the ecosystem depends on the performance of various other assets. Hope for unraveling this complexity is placed in digitalization.
The digital mapping – digital twins – of all essential assets in the system shall prospectively enable the reduction of conservative planning, buffers, and assumptions. This requires integration across the entire ecosystem and, hence, across market participants. Yet, (data) exchange, especially cross-industry, remains a fundamental headache (e.g., IP rights). Operationally, an even greater problem is the lacking consistent, granular taxonomy of the logic, language, and (data) exchange of the "(digital) system train." Consequently, data is often scattered and not systematically collected. Therefore, teaming up across the industry towards an (open) overarching taxonomy and respective framework unifying the different logics, languages, and technologies is key for accelerating the way forward.
The rail operations ecosystem today – a complex puzzle to solve
Does the following scenario sound familiar? Waiting for a late-running train and asking yourself: "What is actually the problem?" Usually, the problem is quite complex, and our late-running train is merely one piece of the overall rail ecosystem puzzle. And this is a tricky puzzle to master: seamlessly integrating all assets involved (e.g., trains, tracks, stations, maintenance) and managing their interdependencies – while continuously monitoring the gap between planned theory and actual reality!
Hope for solving this puzzle is placed in the digitalization of the rail system and its different components. The digital mapping and connection – digital twins – of all essential assets in the system shall generate more and new knowledge about the condition of every single asset and, hence, also about the whole system. Prospectively, this shall reduce conservative planning, buffers, and assumptions, thus increasing capacity, quality, and efficiency. To reach this goal, digitally mapping the ecosystem does not suffice. Data must instead be translated into meaningful conclusions. Only then might the envisioned (quality) improvements in P&L-effective improvements be achieved.
However, the rail ecosystem has grown over many decades. Hence, it is exceptionally heterogeneous today: rolling stock fleets of varying ages and technological standards from different suppliers run on brown- and greenfield networks – but must seamlessly integrate, not only in the real world, but also digitally. This heterogeneity complicates the envisioned digital connection of the rail ecosystem exponentially, due to the following factors, among others:
- Data interpretation: The meaning of train/component data varies not only between different train types, e.g., between high-speed trains and subways or between class 1 and class 2 trains, but also between a particular subway train’s wheelset and that of its neighbor following just one meter behind. Hence, pure AI or data-first approaches often quickly reach their limits, as rail-specific knowledge must be incorporated into these algorithms in order to be able to adapt solutions to respective components (e.g., regarding engineering, technical, physical perspectives).
- Data entanglement: The efficiency of every asset within the ecosystem depends on the performance of many other assets. Consequently, respective digital solutions must map such real-world entanglements between the different assets.
Consequently, to fully scale the industry’s potential, combining and uniformally structuring knowledge about the different assets would appear to be vital.
Digitalizating the entire rail ecosystem as a target
However, while there are many contributors across the entire value chain – from start-ups, traditional suppliers, rolling stock manufacturers, and operators – a core problem remains: The flow of meaningful information between the industry's players is operationally limited, technically tricky, and economically seldom incentive compatible.
The main reasons for such problems may include the following:
- Unclear IP-regulations
Component suppliers, manufacturers, and operators are reluctant to share their data as IP rights and rights of use are not currently clearly regulated. Furthermore, existing platforms are owned and protected by selected competitors.
- Different asset and data logic within the industry
Data is often structured according to different logic. These data structures do not always fit together or do not exist sufficiently granularly, which results in operational problems at the interface between the different market players.
- Inaccurate calculation of total lifecycle costs
Nowadays, operators require a guarantee of maintenance for the total vehicle life cycle. The more accurately OEMs and maintenance providers can forecast future maintenance costs based on data analytics, the more efficient their bids and thus competitiveness.
As a result, the amount of generated data is not the bottleneck for performing purposeful value-adding data analytics, but rather finding a set-up that generates an incentive to exchange and merge data.
In summary, there seems to be a lack of uniform structure – a taxonomy – of the logic, language and, exchange of the "(digital) system train" between the different players.
And, although cross-industry collaboration is gaining pace, a breakthrough has yet to be reached. However, teaming up towards an (open) overarching taxonomy and respective framework unifying the different logics, languages, and technologies could accelerate the way forward. Successful examples of adjacent industries may also encourage the rail industry.
Teaming up to accelerate the digitalization of the rail ecosystem
Developing such a unifying taxonomy is a complex and tedious challenge. It must be concise yet also detailed to the level required. And it should be sufficiently inclusive. The taxonomy should also be comprehensive but remain extendible, allowing for future adaptions and revision. Developing the taxonomy must follow a process of iteratively applying an empirical and deductive approach, using existing industry standards, while further adapting, developing, iterating, and aligning. However, despite these challenges, it will not only support overcoming today's hurdles but also reduce the development cost and pave the way towards a digitally connected rail ecosystem.
In concrete, such taxonomy for a digital rail ecosystem may include the following sub-dimensions:
- Component layer
The different players define the structure of a train in essentially the same way. However, with regard to its granular structure, they define it significantly differently – the first step must therefore be a consistent model of the train, i.e., a logical modeling ("granular taxonomy") systematically and hierarchically of the component groups, i.e., its physical structure.
- Information layer
The information model defines technical, physical connections within and between components that convey data. It manages information flows and interactions between individual components, thus spanning the train's interactions between the individual components.
- Data layer
Along the information nodes defined in the component and information model, a multitude of data is generated, which is recorded in the data layer. The previously spanned network of components and information model is thus "filled with life", and the various events and actions are consolidated. The model includes both generic data (e.g., speed, localization, etc.) and component-specific property data (e.g., the diameter of the wheelset, etc.).
- Interface layer
In the final stage of development, the architecture should define standardized, secure interfaces (APIs) to allow for industry-wide acceptance and adoption – while intellectual property rights (IP) are to be consistently protected.
Hence, the taxonomy and respective framework is a multidimensional construct with different conceptual and technical layers. Combined, the different layers result in the overall structuring framework that represents all relevant dimensions of the rail ecosystem. Building on this institutional framework, the rail ecosystem's intelligence and connection can be built up through various use-cases and simulations.
Author:
Dr. Christian Wältermann, Head of Mobility & Infrastructure (Email)